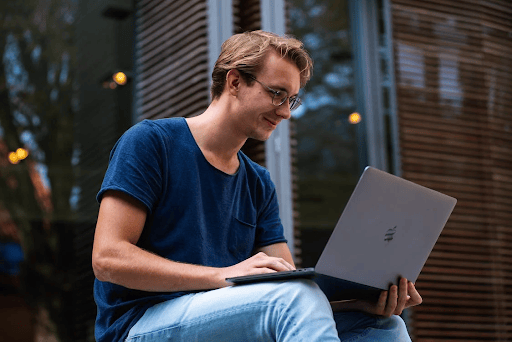
In today’s digital era, 328.77 million terabytes of data are generated daily on average. This vast influx of information encompasses a wide array of sources, ranging from financial transactions and scientific research papers to social media posts and customer reviews.
It can be difficult to make sense of this enormous sea of data and extract useful knowledge from it, but there are priceless insights and patterns waiting to be discovered within it.
Machine learning embeddings offer a practical way to make complicated data easier to understand.
We’ll go into more detail about machine learning embeddings in this blog post, including what they are, how they’re used, and how they can revolutionize data analysis and decision-making.
Understanding Machine Learning Embeddings
At its core, machine learning embeddings provide a representation of data in a lower-dimensional space where similar items are closer together and dissimilar items farther apart.
This transformation occurs through feature learning where an algorithm autonomously extracts relevant features from raw data to form its representation in this lower-dimensional representation.
One of the most widely-used types of embeddings is word embeddings, in which individual words from a corpus are transformed into vectors in continuous space and allow computers to understand the semantic meaning of words based on context; applications include language translation, sentiment analysis, and recommendation systems.
Clustering and Classification
The foundations of machine learning are clustering and classification techniques, which help to simplify complex datasets by either classifying or clustering them together.
Grouping data points with comparable characteristics into coherent clusters using algorithms like K-means or hierarchical clustering reveals latent patterns and structures.
Conversely, classification algorithms like logistic regression or support vector machines allocate labels to data points based on their distinct features, enabling the anticipation of outcomes for new instances. A significant strategy for enhancing data comprehension lies in vector embeddings.
In general, vector embedding can transform high-dimensional data into manageable lower-dimensional representations that preserve semantic relationships while still providing crucial information.
Leveraging their power, machine learning models can easily process and dissect complex datasets for insights that provide informed decision-making as well as drive innovation across diverse domains.
Semantic Similarity
Another compelling aspect of machine learning embeddings is their ability to capture semantic similarity.
Word2vec encodes semantic relationships among words based on the context in large text corpora; similarly, in other fields, embeddings can encode similarity between data points enabling recommendation systems, clustering, or classification tasks to leverage these learned representations more efficiently.
Unsupervised Learning
Many machine learning embedding techniques operate unsupervisedly, meaning they do not rely on labeled data for training purposes. This approach is especially advantageous when labeled data are hard or costly to come by.
Unsupervised embeddings created through autoencoders or GANs capture inherent structures within data that allow unsupervised anomaly detection, visualization, or exploratory data analysis.
Uncovering Hidden Patterns
Machine learning embeddings possess a remarkable capability to unearth concealed patterns and structures within datasets. By leveraging vast quantities of unlabeled data, embeddings illuminate latent relationships that might elude human observation.
This attribute holds particular significance in domains like natural language processing, image recognition, and recommendation systems, where data structures are intricate and multi-faceted.
For instance, in document analysis, embeddings facilitate the clustering of akin documents based on semantic content.
Machine learning embeddings enable tasks like document classification, topic modeling and information retrieval to be carried out more efficiently, leading to improved search outcomes and user interactions.
Through such applications, machine learning embeddings push data analysis further than ever, providing insightful knowledge for informed decision-making.
Enhancing Decision-Making Processes
Machine learning embeddings enable organizations to make better-informed decisions across an array of applications by simplifying complex data.
Embeddings offer critical insights that drive company results and boost competitiveness, from detecting fraudulent activity and personalizing marketing efforts to optimizing supply chains.
Moreover, embeddings can assist healthcare practitioners in utilizing electronic health records to identify individuals who may be at risk of certain medical disorders and initiate early intervention, ultimately leading to better patient outcomes and lower healthcare expenditures.
Challenges and Considerations
While machine learning embeddings hold a lot of promise, they also have a unique set of issues and concerns.
The most important of them is the interpretability of embeddings; their representations can not always line up with human intuition, which makes it challenging to understand why a model makes a particular prediction or suggestion. This can also raise questions about accountability and ethics.
Because embeddings rely heavily on the caliber and volume of training data, thorough preparation and validation are necessary to produce findings that can be trusted.
Furthermore, biases present in training data may amplify through embedding processes leading to unfair or discriminatory results if left unaddressed.
In Closing
Machine learning embeddings offer a revolution in how we understand complex data. By breaking up complex datasets into more manageable representations, embeddings allow us to uncover hidden patterns, streamline decision-making processes, and spark innovation across industries.
Although embeddings may present certain challenges and considerations, their benefits in terms of efficiency, effectiveness, and insight generation cannot be overstated.
Leveraging machine learning embeddings will take us one step closer to realizing data-driven intelligence in digital form.